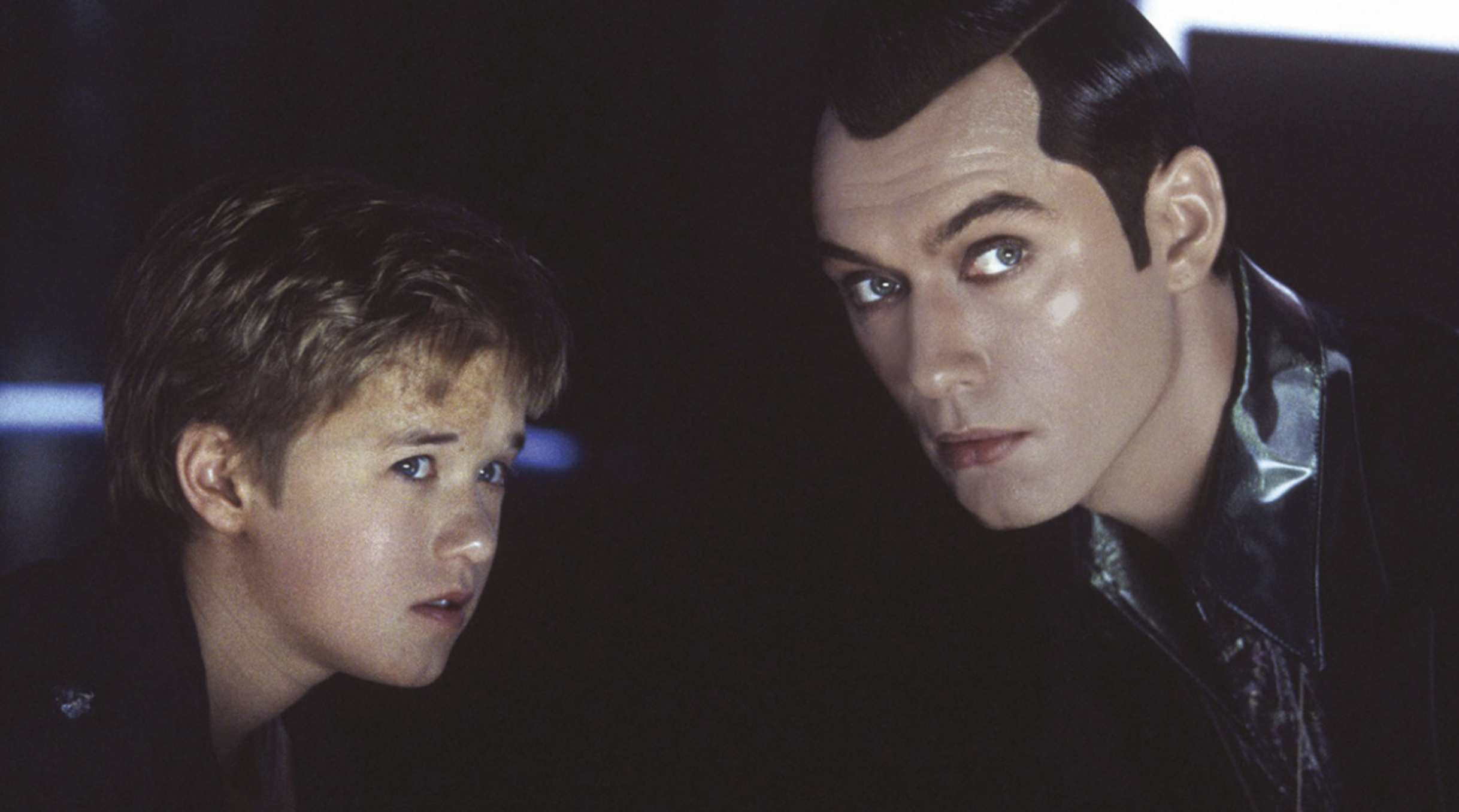
From Spielberg’s A.I. to Fast.ai: My Personal Deep Dive into AI
AI has fascinated me ever since I first watched Spielberg’s A.I. with Haley Joel Osment and Jude Law. I used to watch that movie so much! There was something about the way it blended futuristic technology with deeply human emotions that captured my imagination. The idea that machines could learn, adapt, and even experience emotions got me wondering how far we could actually go with AI in real life. Fast forward to today, and here I am, diving into deep learning—taking the concepts that once seemed so fantastical and exploring their real-world possibilities.
That’s why I decided to jump into deep learning with fast.ai.
Why fast.ai?
I’ve always been a “learn by doing” person, so this course felt perfect. There are tons of other resources out there, but fast.ai clicked because it’s hands-on right from the start. They reference a book called A Mathematician’s Lament, which asks, “What if we taught music the way we teach math? By never playing an instrument.” That hit home for me as someone who loves diving straight in. I need to get my hands dirty and start experimenting.
With deep learning, you’re motivated to improve your model, and that drive makes you dive into the theory. It’s like what I learned in bootcamp—“just in time learning,” where you pick up what you need as you go along. It’s not about cramming all the theory upfront, and that approach works really well for me.
Playfulness and Curiosity
I love this quote from the course:
The people who do well with deep learning tend to have two traits: playfulness and curiosity.
Playfulness and curiosity? Those are basically my middle names.
Of course, learning deep learning isn’t all fun and games. It can be frustrating. You can get stuck…a lot. And when you’re stuck, the best advice is to go back to the last part that made sense and go over it slowly until you figure out what’s tripping you up.
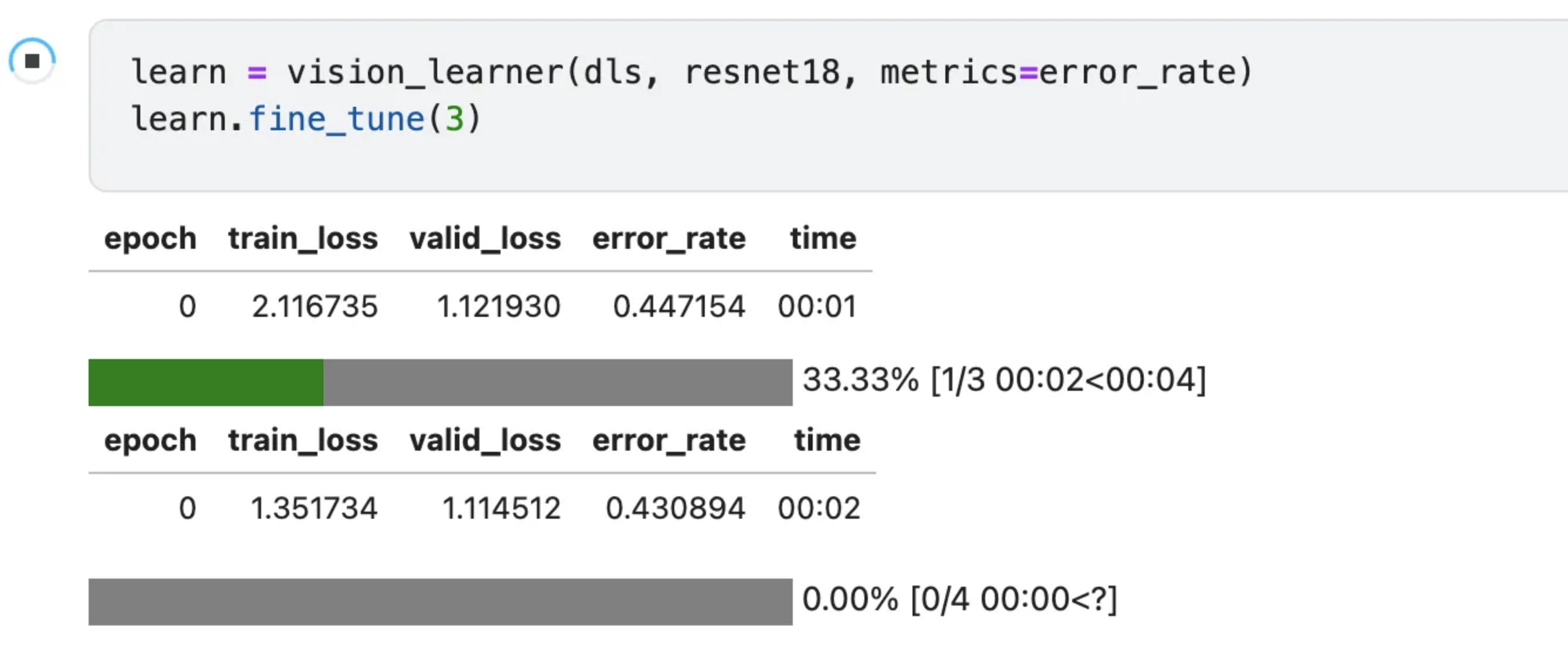
The whole process is a bit of a rush, though. Watching a model train, even slowly, is thrilling. There’s something oddly satisfying about that green progress bar moving along as the model learns. And even though the machine is doing the hard work, I still feel like a genius for being involved.
Having Fun with Models
Naturally, I had some fun with it. I ran some experiments—like, could the model tell the difference between a ghost and a human? Now, I know this isn’t exactly a scientific model (spoiler: ghosts aren’t really data-friendly subjects!), but I figured it would be a fun way to play around and see how the model responds to unusual inputs. Of course, most “ghost” photos are likely just humans or blurry objects anyway. Then there was the classic Silicon Valley moment—hot dog or pizza? The results were a bit of a mess, probably because I left in random parameters like “shade” and “sun” when they didn’t really apply to the training data. But hey, that is a hot dog, right?
What this playful approach made me realize is how critical good data is. Models are only as smart as the data they learn from, and if that data isn’t clean, relevant, or diverse, the results can get skewed. In this case, my random parameters and inconsistent data were reflected in the messy predictions. It’s a good reminder that feeding the model accurate and high-quality data is key to getting meaningful results. It’s something I’m definitely keeping in mind as I move forward.
Reflecting on My First Few Weeks
Looking back on my first couple of weeks diving into deep learning, it’s been a mix of excitement and frustration. Excitement when I see things work (even if it’s just distinguishing a hot dog from pizza), and frustration when the model takes forever to train, or when I hit a wall and have to backtrack. But the thing is, it’s all part of the process. I’m learning to be more playful, more curious, and—most importantly—more patient with myself.
What’s funny is how much this journey reminds me of the themes from A.I. that sparked my interest in the first place. Back then, I was captivated by the idea of machines learning, evolving, and even developing their own forms of intelligence. Now, I’m exploring that very concept firsthand. The futuristic ideas that once seemed like pure imagination are now part of my reality, and it’s both challenging and incredibly rewarding.
While I’m not building robots like the ones in the movie, what I am doing is laying the groundwork for understanding how machines can learn. And honestly, that’s enough to keep me going. The more I learn, the more I realize there’s so much more to explore, and I can’t wait to dive even deeper into this world that once felt like science fiction.